 | 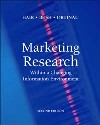 Marketing Research: Within a Changing Information Environment, 2/e Joseph Hair,
Louisiana State University Robert Bush,
University of Memphis David Ortinau,
University of South Florida
Data Analysis: Testing for Association
Chapter SummaryUnderstand and evaluate the types of relationships between variables. Relationships between variables can be describes in several ways, including presence of a relationship, direction, strength of association and type of relationship. We will describe each of these concepts. Presence tells us whether a consistent and systematic relationship exists while direction tells us whether the relationship is positive or negative. Strength of association tells us whether we have a weak or strong relationship, and the type of relationship is usually described as either linear or nonlinear. Two variables may share a linear relationship, in which changes in one variable are accompanies by some change (not necessarily the same amount of change) in the other variable. As long as the amount of change stays constant over the range of both variables, the relationship is termed linear. Relationships between two variables that change in strength and/or direction as the values of the variables change are referred to as curvilinear.
Understand the concept of association and covariaton. The terms covariation and association refer to the attempt to quantify the strength of the relationship between two variables. Covariation is the amount of change in one variable of interest that is consistently related to change in another variable under study. The degree of association is a numerical measure of the strength of the relationship between two variables. Both these terms refer to linear relationships.
Understand the differences in Chi Square, Pearson Correlation, and Spearman Correlation. The chi-square statistic permits us to test for significance between the frequency distributions of two or more groups. Categorical data from questions about gender, race, profession, and so forth can be examined and tested for statistical differences. Pearson correlation coefficients are a measure of linear association between two variables of interest. The Pearson correlation coefficient is used when both variables are measured on an interval or ratio scale. When one or more variables of interest are measured on an ordinal scale, the Spearman rank order correlation coefficient should be used.
Explain the concept of practical significance versus statistical significance. Because some of the procedures involved in determining the statistical significance of a statistical test include consideration of the sample size, it is possible to have a very low degree of association between two variables show up as statistically significant (i.e., the population parameter is not equal to zero). However, by considering the absolute strength of the relationship in addition to its statistical significance, the research is better able to draw the appropriate conclusion about the data and the population from which they were selected.
Understand when and how to use regression analysis. Regression analysis is useful in answering questions about the strength of a linear relationship between a dependent variable and one or more independent variables. The results of a regression analysis indicate the amount of change in the dependent variable that is associated with a one unit change in the independent variables. In addition, the accuracy of the regression equation can be evaluated by comparing the predicted values of the dependent variable to the actual values of the dependent variable drawn from the sample.
|
|