 | 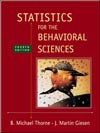 Statistics for the Behavioral Sciences, 4/e Michael Thorne,
Mississippi State University -- Mississippi State Martin Giesen,
Mississippi State University -- Mississippi State
Two-Way Analysis of Variance
Chapter OverviewThe two-way ANOVA is presented in this chapter as a method for analyzing data resulting from the
administration of two or more levels of two independent variables. When there is more than one
independent variable, the variables are called factors.
In a two-factor experiment, the effect of each separate independent variable is called a main effect.
Thus, a two-factor experiment has two main effects. Also, there is the possibility of interaction or the joint
effect of two independent variables on behavior. If an interaction effect exists, the effect of one factor
depends on the levels of the second factor.
One way to look for interaction or the lack of it is to graph the results of a factorial study. If there is no
interaction, the graph will show roughly parallel lines or lines that are approximately equidistant at each
data point. Interaction is shown by converging or crossing lines. “Rules” for interpreting graphs of two-factor experiments are as follows:- Assuming factor A is shown on the baseline and the levels of factor B are plotted as different lines, if the averages of the points above each level of factor A are unequal, there may be a significant main effect for factor A.
- If the averages of the points used to plot the lines are unequal, there may be a significant main effect for factor B.
- If the lines converge, cross, or in some way depart from parallel, there may be a significant interaction.
The main advantage of the two-factor design is that you can test for interaction between the factors. If
you manipulated the same variables in two separate one-factor experiments, you would not be able to test
for an interaction of the factors. Another advantage of the two-factor design is that it allows a savings in the
number of subjects, and probably the time and resources, needed. The two-factor design usually increases
the power of the tests on the main effects and permits greater generalizability.
The logic of the tests for two-factor designs is the same as for the one-way ANOVA. Instead of one F
test, there are three F tests for the two-way ANOVA: main effects tests for factors A and B, and a test of
the interaction between factors A and B. Each F ratio consists of the variance (MS) for each effect divided
by the variance within treatments. The same error term, MSw, is used for each F ratio. The variance
between treatments is assumed to be caused by individual differences, experimental error, and a treatment
effect; the variance within treatments is caused by individual differences and experimental error. An F ratio
close to 1.00 indicates the lack of a treatment effect, and a value much larger than 1.00 signals a valid
treatment effect.
Interpretation of the two-way ANOVA depends in large part on whether the interaction is significant.
If the interaction is not significant, significant main effects can be analyzed with the post hoc tests
discussed in Chapter 11. The first step in interpreting a significant interaction is to plot the group means,
with further interpretation requiring individual group comparisons. |
|