 | 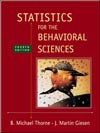 Statistics for the Behavioral Sciences, 4/e Michael Thorne,
Mississippi State University -- Mississippi State Martin Giesen,
Mississippi State University -- Mississippi State
Confidence Intervals and Hypothesis Testing
Chapter OverviewThe sampling distribution of means is derived by taking successive, same-sized random samples from some
population, computing a mean for a measurable characteristic of each sample, and plotting the means on a
frequency polygon. The first property of the sampling distribution is that its mean is the mean of the
population or µ. The second property, which is a simplified version of the central limit theorem, is that the
larger the size of each sample, the more nearly the sampling distribution will approximate the normal
curve. The third property is that the larger the sample size, the smaller the standard deviation of the
sampling distribution. The standard deviation is called the standard error of the mean.
When population parameters (µ and σ) are known, z scores are appropriate. However, we often do not
know them, so they must be estimated from sample values. The sample mean ( (0.0K) ) is an unbiased estimate
of µ, and, as we defined it in Chapter 6, sample variance (s2) is an unbiased estimate of population variance
(σ2). Recall that to obtain an unbiased estimate of σ2, we divided the sum of squared deviations by N - 1
rather than by N. This expression, N - 1, is called degrees of freedom and is defined as the number of
values free to vary after certain restrictions (e.g., the sum of the deviations equals 0) are placed on the data.
A confidence interval is a range of values within which the population mean almost certainly lies. The
confidence intervals usually computed are the 95% and the 99% confidence intervals. Equations for the
confidence intervals are derived in the chapter from the formula introduced in Chapter 6 to convert z scores
to raw scores.t scores are estimated z scores. They are used in place of z scores when population parameters are
estimated from the sample. t scores correspond to the t distribution, and the t scores used in the confidence
interval equations are determined from Table B (see Appendix 2), which contains values of t cutting off
deviant portions of the distribution. In order to use the table of critical t scores, we need to know the df. For
confidence intervals and the one-sample t test, df = N - 1. A confidence interval is an interval estimate of
the population mean.
Another important use of the distribution of t is to test hypotheses about the value of µ. The seven-step
procedure introduced for testing the null hypothesis is as follows:
- State the null hypothesis in symbols (H0: µ = µ0) and in words in the context of the problem.
- State the alternative hypothesis in symbols (e.g., H1: µ ≠ µ0) and in words.
- Choose an a level, the level at which you will reject or fail to reject the null hypothesis. Set a = .05, if there are no specific instructions in the problem.
- State the rejection rule. For example, the rule for a particular problem may be as follows: If |tcomp| is ≥ tcrit, then reject the null hypothesis. This is the rejection rule for a nondirectional hypothesis.
- Compute the test statistic. In this chapter, the equation for the test statistic is
 (1.0K) - Make a decision by applying the rejection rule.
- Write a conclusion statement in the context of the problem.
For a directional test, H0 is rejected if tcomp is of the same sign but is more extreme than tcrit.
A Type I, or a, error (a "false claim") is defined as rejecting the null hypothesis when it is really true.
The probability of an a error is equal to a, the level at which we are trying to reject the null hypothesis.
Failing to reject H0 when it is false is called a Type II, or β error (a "failure of detection"). Lowering
the value of a increases the probability of a β error.
The power of a statistical test is the probability that the test will detect a false null hypothesis. Factors
affecting the power of a test are
- The size of a. The smaller the a level, the less powerful the test will be.
- The sample size. The larger the sample size, the greater the power of the test will be.
- The distance between the hypothesized mean and the true mean. The greater the distance, the greater the power of the test will be.
In analyzing the results from large numbers of research studies, meta-analysis uses quantitative
procedures to integrate the findings. Meta-analysis uses the effect size - the size of the difference between
the null hypothesis and the alternative hypothesis in standardized units - results of studies rather than
simply reporting whether or not the results were statistically significant.
Some researchers argue that hypothesis testing should be abandoned, claiming that the procedures are
misleading and we should instead report confidence intervals and effect sizes. People opposed to
hypothesis testing hold that many research studies don't have enough power to find what they are looking
for, with a corresponding increase in Type II errors. This point is valuable if it forces experimenters to be
more attentive to having sufficient power to detect an effect if it is present. |
|